The unhappy marriage of data stacks, modernity, and capital letters
The data industry has a naming problem.
I mostly write (or plan to) about Data orgs, technology, metrics, and some miscellaneous work-related stuff, and publish a new post every few weeks. If you want to read more of my work, please subscribe below. Thanks for reading!
A couple of years ago, during my time at Impala, a debate was underway on Slack about the relative merits of Loom and CloudApp, two screen-recording platforms. While my opinion of CloudApp was always going to be *ahem* clouded by the fact that I could never get it to work, the biggest complaint I had was about the name.
So, we’ve built this app that records stuff on your screen. The really neat thing about this app though is that, rather than saving the recording locally on your machine, it stores it in the cloud, so you can easily share it with other people in your organisation without having to attach huge files to Slack messages and emails. But what name could possibly do justice to such a concept? An app that records your screen and stores the video files in the cloud? Let’s call it… um… Cloud… App.
Whatever your opinion of literal product names (e.g. Hotels.com, Webuyanycar.com, Shit Shirts), “CloudApp” is literal in a meaningless way. You could give the same name to thousands of apps, serving a multitude of use cases, that utilise cloud technology in some way, and the name would make an equal amount of sense in all cases. The fact that it is an app that leverages the cloud is of no significance. A meaningfully descriptive name (if that is indeed the aim) would focus on the screen capture/recording element, and not the cloud element.
I often have similar feelings about the term that we’ve collectively come to use to describe a popular set of software products that are used to ingest, transform, store, analyse, and monitor business data: the Modern Data Stack.
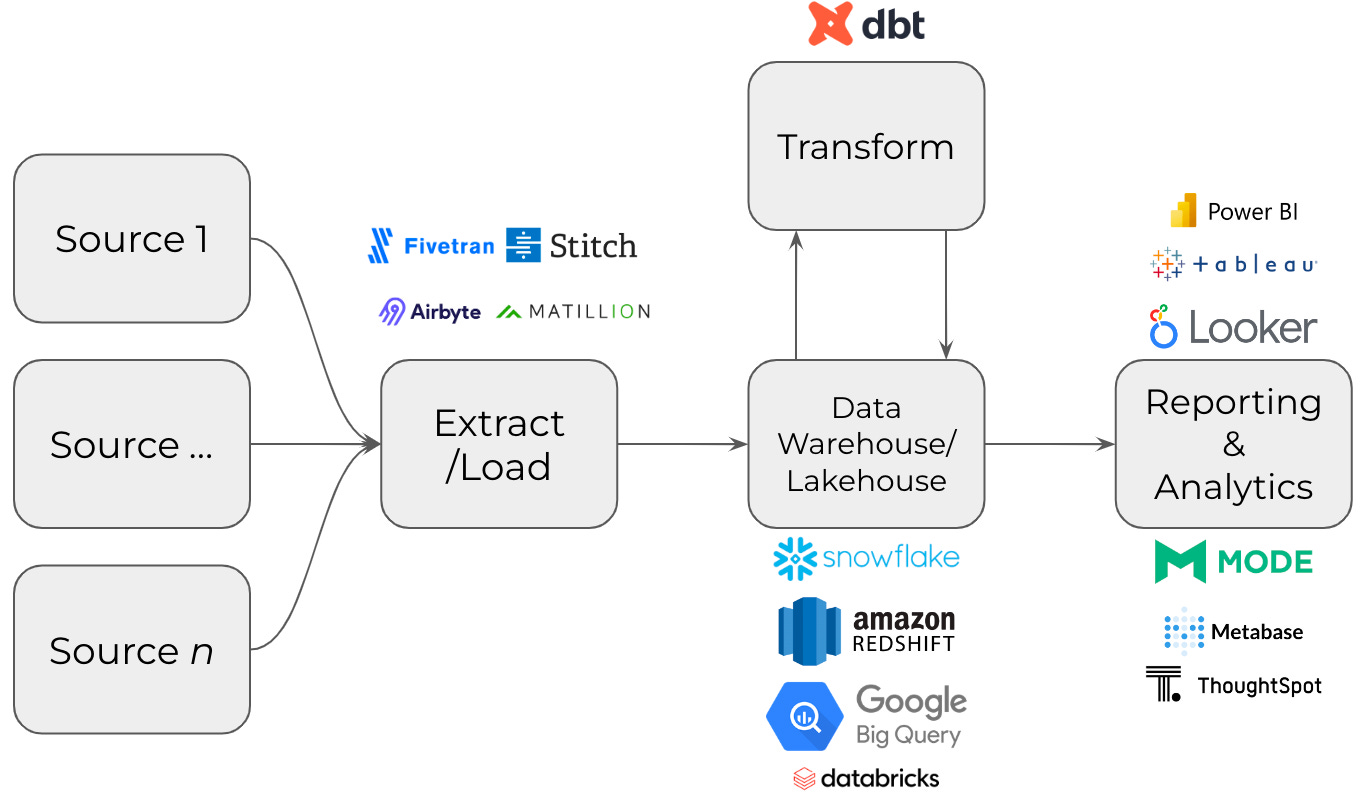
A few days ago, I asked the Mastodon Data community for help tracking down the origin of the term “Modern Data Stack”.
While nobody, it seems, is quite sure where it came from, based on a mere two sources there is some reason to believe that the term originated from Fivetran. The evidence isn’t exactly watertight. One source - an episode of the Data Radicals podcast - credits Taylor Brown (COO of Fivetran) with “popularising” the term, while the other (credit to Josh Wills for finding it) is a 2017 Fivetran blog post referencing the “Modern Data Pipeline”.
Even ChatGPT wasn’t able to help.
Regardless of who deserves the credit/blame for its now pervasive use, the word “modern” seems pretty useless in this context. While we might consider anything at the forefront of technology in the present day to be modern, the state of being modern is not an innate characteristic of the currently-popular ecosystem of data tools. As I wrote in an earlier post, modernity is a transient state, and tools come and go. There was a time when Hadoop was modern. Didn’t that go well?
It’s debatable whether an ecosystem of software products that happen to be popular at a certain point in time even needs a name, but if we’re to concede that it does, then a name that actually describes what’s special or interesting about the thing in question would surely be more appropriate. In ten years’ time, I’m sure, we’ll all look back and laugh at the primitive data tools of 2023 and their dated UIs. In ten years’ time, we’ll realise, those tools of yesteryear were not modern. It’s today’s tools that are modern.
Rather than coalesce around a descriptive name, we have instead coalesced around a name that implies judgment. The implication of the word “modern” is that the approach of using a multitude of SaaS products to turn raw data into insights is inherently more advanced than the methods and tools of earlier decades. Surely, only a chimpanzee would use Teradata? Surely, only a fricking dinosaur - an absolute diplodocus of the data world - would transform their data before loading it to their warehouse, rather than afterwards?
This might seem harmless. It’s only a name, after all, and if it gives people a useful shorthand for something that would otherwise take much longer to say, then that’s all well and good. The problem is that words matter. The MDS does not necessarily represent the best approach for every modern use case, and it seems disingenuous to promote the notion that it does.
The cynic in me thinks that this is all just a ruse to sell more software.1 I have no data to back this up (maybe because my attempts at finding and wrangling this data just weren't modern enough), but I think it's a reasonable bet that Fivetran, dbt Labs, Snowflake et al. have benefited (perhaps beyond their due, in some cases) from the positioning of their products as indispensable nodes on the only data pipeline graph that's considered socially acceptable today.
Worse still, the “Modern Data Stack” has become a proper noun. In reverence to the gods of separated storage and compute, we have somehow reached the point where we assign it capital letters. Even the acronym MDS is widely used, and scarcely anybody bats an eyelid.
What I find most disappointing about the “Modern Data Stack” (the name, not the set of tools) is that it implies a lack of ambition. It suggests that the philosophy and tools that it describes are the last word in data management. The March of Progress has reached its final point, and we can now look back on our short, stupid, hunchbacked ancestors with contempt. The evocation of modernity, combined with grandiose capitalisation, lends the so-called Modern Data Stack a grim air of inevitability.
Some, of course, do not accept the finality of the Modern Data Stack. Some have made reference to a “Post-Modern Data Stack”, thus pushing data industry commentary ever closer to the genre of farce.
For all its promise, I’m not sure that there’s any consensus over what the PMDS actually is. According to one source, it’s our friendly neighbourhood MDS tools with some MLOps practices thrown in; according to another, it’s our friendly neighbourhood MDS tools but with better cataloguing, observability, and communication. Whatever is meant by the Post-Modern Data Stack, my only confident prediction is that The People Who Give Names To Things are going to have a problem on their hands when the industry inevitably comes up with something that supersedes the things that they’ve hitherto referred to as “post-modern”.
Reading the above, you’d be forgiven for thinking that I have an axe to grind with the Modern Data Stack (the tools themselves, not the name). I don’t.
Whether it’s Fivetran, dbt, Snowflake, Looker, Dagster, or any other competing or complementary tool, each one serves a purpose. In most cases, each tool fulfils a single role in the data pipeline, and does it well. This is broadly in keeping with the first tenet of the Unix philosophy, developed several decades ago and still embraced by software engineers today. It’s also a good thing for competition. In most cases, it doesn’t matter whether you host your platform on AWS, GCP or Azure: your choice of data management software (except for your warehouse) usually won’t be limited by this, or by the other tools that you use elsewhere in the pipeline. Most of them are interoperable and cloud platform-agnostic.
In some ways, the Modern Data Stack is arguably more modern than what came before. In the same way that one can use “modern” telecommunications technology to reach somebody on the other side of the planet instantaneously, it’s now relatively trivial for somebody with some SQL and a company credit card to use “modern” data tools to stand up a data stack that gets data from source to business stakeholder in a basically acceptable manner, without help from anybody else. This was much less straightforward a decade ago.
Of course, reducing barriers to entry comes at a cost. While the MDS enables those with a traditional Data Analyst skill set to build well-functioning ELT processes (where in years gone by this would have required the skills of a Data Engineer), this doesn’t necessarily mean that the outcomes are always better.
As powerful and time-saving as tools such as Fivetran and dbt may be, if used recklessly the costs can spiral out of control. Likewise, dbt’s democratisation of data transformation and modelling, combined with cheap cloud storage, has arguably led the practice of data modelling astray, to the point where companies with clumsy, Wild West implementations of the MDS are now dealing with messy, duplicative, inconsistent data models, and the hefty compute costs that often arise as a result.
In any case, while the perception of the MDS as “modern” by today’s standards is understandable, this doesn’t make the name any less silly. So, what would I call it instead?
At this point, it doesn’t really matter. That horse has bolted, and it’s unlikely that an alternative name will gain traction. If we could turn back time though, one might advocate for the Modular Data Stack as a more descriptive name that’s less likely to be rendered meaningless by time, and doesn’t give software vendors quite so much emotional sway. It’s not such a leap either. The acronym remains the same, and even the first three letters don’t have to change. All we have to get used to is saying “ular” instead of “ern”. Is that really too much to ask?
I would argue, though, that modularity is not necessarily the salient characteristic of the thing(s) that we call the Modern Data Stack. Rather, the elements that I see as most important are the effectively infinite scaling of computing power (decoupled from storage capacity), the interoperability of non-vertically integrated software, and the facilitation of the return to SQL as the primary language for data management.
With that in mind, I propose that we begin calling it the Modular Interoperable SQL-Led Infinitely Scaling Horizontally Integrated Data Stack (MISLISHIDS for short). Simple. Has a nice modern ring to it too.
The optimist in me agrees, but would perhaps replace the word “ruse” with “branding exercise”.